建议在网络上广为流传。
- “买了那件东西?试试这些类似的项目。
- “喜欢那本书吗?试试这些类似的标题。
- “不是您要找的帮助页面?试试这些类似的页面。
此笔记本演示如何使用嵌入来查找要推荐的类似项目。特别是,我们使用 AG 的新闻文章语料库作为我们的数据集。
我们的模型将回答这个问题:给定一篇文章,还有哪些文章与它最相似?
1 2 3 4 5 6 7 8 9 10 11 12 |
import pandas as pd import pickle from embeddings_utils import ( get_embedding, distances_from_embeddings, tsne_components_from_embeddings, chart_from_components, indices_of_nearest_neighbors_from_distances, ) EMBEDDING_MODEL = "text-embedding-3-small" |
1. 加载数据
接下来,让我们加载 AG 新闻数据,看看它是什么样子的。
openai-cookbook/examples/data/AG_news_samples.csv at main · openai/openai-cookbook (github.com)
1 2 3 4 5 6 |
# load data (full dataset available at http://groups.di.unipi.it/~gulli/AG_corpus_of_news_articles.html) dataset_path = "data/AG_news_samples.csv" df = pd.read_csv(dataset_path) n_examples = 5 df.head(n_examples) |
运行结果:
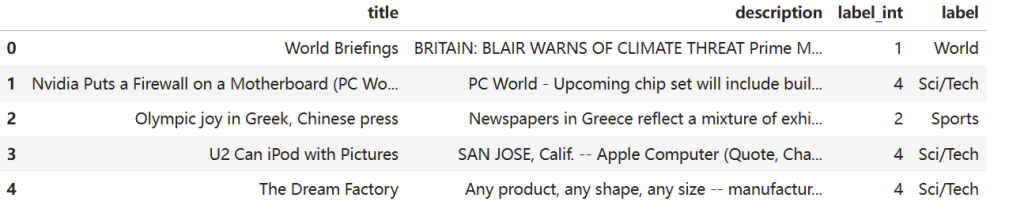
让我们看一下这些相同的示例,但没有被省略号截断。
1 2 3 4 5 6 |
# print the title, description, and label of each example for idx, row in df.head(n_examples).iterrows(): print("") print(f"Title: {row['title']}") print(f"Description: {row['description']}") print(f"Label: {row['label']}") |
运行结果:
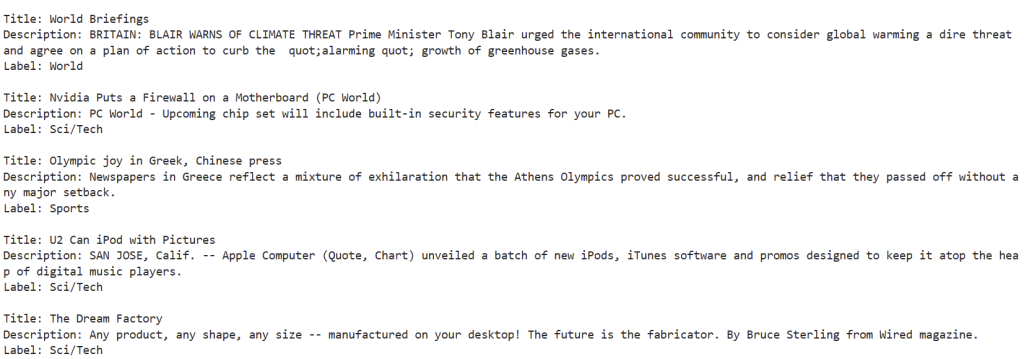
2. 构建缓存以保存嵌入
在获取这些文章的嵌入之前,让我们设置一个缓存来保存我们生成的嵌入。通常,最好保存嵌入内容,以便以后可以重复使用它们。如果您不保存它们,则每次再次计算它们时都会再次付款。
缓存是一个字典,它将元组(text, model)
映射到嵌入,嵌入是浮点数的列表。缓存保存为 Python pickle 文件。
1 2 3 4 5 6 7 8 9 10 11 12 13 14 15 16 17 18 19 20 21 22 23 24 25 26 |
# establish a cache of embeddings to avoid recomputing # cache is a dict of tuples (text, model) -> embedding, saved as a pickle file # set path to embedding cache embedding_cache_path = "data/recommendations_embeddings_cache.pkl" # load the cache if it exists, and save a copy to disk try: embedding_cache = pd.read_pickle(embedding_cache_path) except FileNotFoundError: embedding_cache = {} with open(embedding_cache_path, "wb") as embedding_cache_file: pickle.dump(embedding_cache, embedding_cache_file) # define a function to retrieve embeddings from the cache if present, and otherwise request via the API def embedding_from_string( string: str, model: str = EMBEDDING_MODEL, embedding_cache=embedding_cache ) -> list: """Return embedding of given string, using a cache to avoid recomputing.""" if (string, model) not in embedding_cache.keys(): embedding_cache[(string, model)] = get_embedding(string, model) with open(embedding_cache_path, "wb") as embedding_cache_file: pickle.dump(embedding_cache, embedding_cache_file) return embedding_cache[(string, model)] |
让我们通过嵌入来检查它是否有效。
1 2 3 4 5 6 7 |
# as an example, take the first description from the dataset example_string = df["description"].values[0] print(f"\nExample string: {example_string}") # print the first 10 dimensions of the embedding example_embedding = embedding_from_string(example_string) print(f"\nExample embedding: {example_embedding[:10]}...") |
运行结果:

3. 基于嵌入推荐类似文章
要查找类似的文章,让我们遵循三步计划:
- 获取所有文章描述的相似性嵌入
- 计算源标题与所有其他文章之间的距离
- 打印出最接近源标题的其他文章
1 2 3 4 5 6 7 8 9 10 11 12 13 14 15 16 17 18 19 20 21 22 23 24 25 26 27 28 29 30 31 32 33 34 35 36 37 38 39 40 41 42 |
def print_recommendations_from_strings( strings: list[str], index_of_source_string: int, k_nearest_neighbors: int = 1, model=EMBEDDING_MODEL, ) -> list[int]: """Print out the k nearest neighbors of a given string.""" # get embeddings for all strings embeddings = [embedding_from_string(string, model=model) for string in strings] # get the embedding of the source string query_embedding = embeddings[index_of_source_string] # get distances between the source embedding and other embeddings (function from utils.embeddings_utils.py) distances = distances_from_embeddings(query_embedding, embeddings, distance_metric="cosine") # get indices of nearest neighbors (function from utils.utils.embeddings_utils.py) indices_of_nearest_neighbors = indices_of_nearest_neighbors_from_distances(distances) # print out source string query_string = strings[index_of_source_string] print(f"Source string: {query_string}") # print out its k nearest neighbors k_counter = 0 for i in indices_of_nearest_neighbors: # skip any strings that are identical matches to the starting string if query_string == strings[i]: continue # stop after printing out k articles if k_counter >= k_nearest_neighbors: break k_counter += 1 # print out the similar strings and their distances print( f""" --- Recommendation #{k_counter} (nearest neighbor {k_counter} of {k_nearest_neighbors}) --- String: {strings[i]} Distance: {distances[i]:0.3f}""" ) return indices_of_nearest_neighbors |
4. 示例建议
让我们寻找与第一篇类似的文章,这是关于托尼·布莱尔的。
1 2 3 4 5 6 7 |
article_descriptions = df["description"].tolist() tony_blair_articles = print_recommendations_from_strings( strings=article_descriptions, # let's base similarity off of the article description index_of_source_string=0, # articles similar to the first one about Tony Blair k_nearest_neighbors=5, # 5 most similar articles ) |
运行结果:
挺好的!5项建议中有4项明确提到了托尼·布莱尔,第五项是伦敦关于气候变化的文章,这些话题可能经常与托尼·布莱尔有关。
让我们看看我们的推荐者在第二篇关于NVIDIA新芯片组的示例文章中的表现,该芯片组具有更高的安全性。
1 2 3 4 5 |
chipset_security_articles = print_recommendations_from_strings( strings=article_descriptions, # let's base similarity off of the article description index_of_source_string=1, # let's look at articles similar to the second one about a more secure chipset k_nearest_neighbors=5, # let's look at the 5 most similar articles ) |
运行结果:
从打印的距离中,您可以看到 #1 推荐比其他所有推荐更接近(0.11 与 0.14+)。#1 建议看起来与起始文章非常相似 – 这是 PC World 关于提高计算机安全性的另一篇文章。挺好的!
附录:在更复杂的推荐器中使用嵌入
构建推荐系统的一种更复杂的方法是训练机器学习模型,该模型接收数十或数百个信号,例如项目受欢迎程度或用户点击数据。即使在这个系统中,嵌入也可以成为推荐器中非常有用的信号,特别是对于尚未“冷启动”且尚未获得用户数据的项目(例如,在没有任何点击的情况下添加到目录中的全新产品)。
附录:使用嵌入可视化类似文章
为了了解我们最近的邻居推荐器在做什么,让我们可视化文章嵌入。虽然我们无法绘制每个嵌入向量的 2048 个维度,但我们可以使用 t-SNE 或 PCA 等技术将嵌入压缩为 2 或 3 个维度,我们可以绘制这些维度。
在可视化最近邻之前,让我们使用 t-SNE 可视化所有文章描述。请注意,t-SNE 不是确定性的,这意味着结果可能因运行而异。
1 2 3 4 5 6 7 8 9 10 11 12 13 14 15 |
# get embeddings for all article descriptions embeddings = [embedding_from_string(string) for string in article_descriptions] # compress the 2048-dimensional embeddings into 2 dimensions using t-SNE tsne_components = tsne_components_from_embeddings(embeddings) # get the article labels for coloring the chart labels = df["label"].tolist() chart_from_components( components=tsne_components, labels=labels, strings=article_descriptions, width=600, height=500, title="t-SNE components of article descriptions", ) |
如上图所示,即使是高度压缩的嵌入也能很好地按类别对文章描述进行聚类。值得强调的是:这种聚类是在不了解标签本身的情况下完成的!
此外,如果您仔细观察最令人震惊的异常值,它们通常是由于标记错误而不是嵌入不良造成的。例如,绿色运动聚类中的大多数蓝色世界点似乎是体育故事。
接下来,让我们根据它们是源文章、其最近的邻居还是其他来重新着色这些点。
1 2 3 4 5 6 7 8 9 10 11 12 13 14 15 16 17 18 |
# create labels for the recommended articles def nearest_neighbor_labels( list_of_indices: list[int], k_nearest_neighbors: int = 5 ) -> list[str]: """Return a list of labels to color the k nearest neighbors.""" labels = ["Other" for _ in list_of_indices] source_index = list_of_indices[0] labels[source_index] = "Source" for i in range(k_nearest_neighbors): nearest_neighbor_index = list_of_indices[i + 1] labels[nearest_neighbor_index] = f"Nearest neighbor (top {k_nearest_neighbors})" return labels tony_blair_labels = nearest_neighbor_labels(tony_blair_articles, k_nearest_neighbors=5) chipset_security_labels = nearest_neighbor_labels(chipset_security_articles, k_nearest_neighbors=5 ) |
1 2 3 4 5 6 7 8 9 10 |
# a 2D chart of nearest neighbors of the Tony Blair article chart_from_components( components=tsne_components, labels=tony_blair_labels, strings=article_descriptions, width=600, height=500, title="Nearest neighbors of the Tony Blair article", category_orders={"label": ["Other", "Nearest neighbor (top 5)", "Source"]}, ) |
看看上面的2D图表,我们可以看到关于托尼·布莱尔的文章在世界新闻集群中有些接近。有趣的是,尽管 5 个最近邻(红色)在高维空间中最接近,但它们并不是这个压缩 2D 空间中最近的点。将嵌入压缩到 2 维会丢弃它们的大部分信息,并且 2D 空间中最近的邻居似乎不如完整嵌入空间中的邻居重要。
1 2 3 4 5 6 7 8 9 10 |
# a 2D chart of nearest neighbors of the chipset security article chart_from_components( components=tsne_components, labels=chipset_security_labels, strings=article_descriptions, width=600, height=500, title="Nearest neighbors of the chipset security article", category_orders={"label": ["Other", "Nearest neighbor (top 5)", "Source"]}, ) |
对于芯片组安全示例,完整嵌入空间中的 4 个最近邻在此压缩的 2D 可视化中仍然是最近邻。第五个显示得更远,尽管在整个嵌入空间中更近。
如果需要,还可以使用函数 chart_from_components_3D
制作嵌入的交互式 3D 图。(这样做需要使用n_components=3
重新计算 t-SNE 组件。